Data Quality Metrics You Should Track and Measure
Flipnode on Jun 08 2023
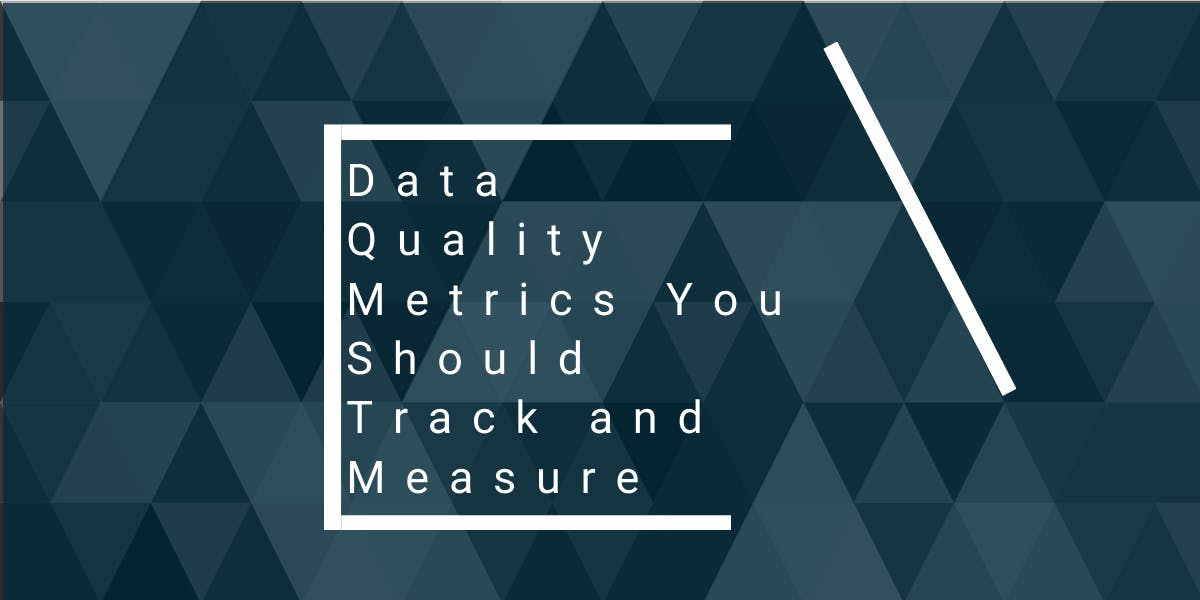
The detrimental effects of low-quality data cannot be underestimated, as it hampers revenue opportunities and impairs sound business decision-making. According to IBM's research, poor data quality results in an annual loss of $3.1 trillion for businesses in the United States alone. The impact goes beyond financial implications, as it also consumes valuable time, leads to customer dissatisfaction, and hinders employee performance and satisfaction.
Given these challenges, it is crucial to have an effective approach for tracking and accessing collected public data to ensure its utmost quality. A few months ago, Allen O'Neill highlighted the significance of maintaining data consistency in his insightful guest post on our blog. Today, we aim to delve deeper into this subject by exploring essential data quality metrics that every business should monitor and evaluate.
Why is data quality important?
The importance of data quality can be summarized quite simply: the higher the quality of your data, the greater the benefits you can derive from it. In essence, data quality is vital because it enables businesses to access accurate and timely public information, facilitating effective service management and resource utilization.
Some potential benefits of high-quality data include:
- Simplified data analysis and implementation
- Informed decision-making based on reliable insights
- Enhanced understanding of customers' needs
- Improved marketing strategies for targeted outreach
- Competitive advantage through data-driven operations
- Increased profitability resulting from optimized processes
What are the 6 dimensions of data quality?
Now that we have gained a comprehensive understanding of the significance of data quality, let's delve into each of the data quality dimensions that collectively define the overall value of collected public information.
Organizations universally recognize that data quality can be categorized into six core dimensions:
Completeness:
Completeness refers to the presence of all the required information in a dataset. For example, when a customer makes a purchase on an online store, the shipping information must be provided completely to ensure accurate delivery.
Accuracy:
Data accuracy reflects how well the collected public information represents the real world. To assess accuracy, consider questions such as, "Does the data accurately depict the actual situation?" and "Are there any incorrect or erroneous data that need to be addressed?"
Consistency:
Maintaining consistency across different data storage locations is crucial to ensure high-quality data. Even a minor discrepancy between two records can jeopardize the integrity and reliability of the data.
Validity:
Validity measures how well data conforms to the required value attributes. For instance, if a date is entered in a format different from the specified format on a platform or website, the data is considered invalid. Validity can be assessed by checking if the information adheres to predefined formats or business rules.
Timeliness:
Timeliness refers to the currency and up-to-dateness of information. If specific public data was collected a year ago and new insights have emerged since then, the data may be considered outdated and in need of updating. Timeliness also encompasses the prompt availability of data to stakeholders, ensuring it can be used in a timely manner.
Uniqueness:
Uniqueness indicates that information appears only once in the database. Since data duplication is not uncommon, it is vital to review the data and eliminate any redundant entries to meet the requirements of this dimension.
To effectively track and measure data quality, it is essential to define specific and measurable metrics for each dimension. These metrics provide a quantifiable assessment of data quality and enable organizations to continuously monitor and improve the quality of their data.
Data quality metrics you should measure and track
Indeed, understanding the dimensions of data quality may appear relatively straightforward. However, possessing this knowledge alone is insufficient for effectively tracking and measuring the quality of your data. To achieve that, the six dimensions should be translated into specific and measurable metrics, often referred to as database quality metrics or objective data quality metrics.
What sets data quality dimensions apart from data quality metrics?
While dimensions provide a general understanding of their importance, data quality metrics offer precise means of measuring and tracking each dimension over time.
For example, when considering the completeness dimension, a common metric could be the number of empty values. This metric helps indicate the extent of missing or misplaced information within the dataset.
Regarding the accuracy dimension, a prominent data quality metric is the ratio of data to errors. This metric enables businesses to monitor the number of incorrect entries, such as missing or incomplete values, in relation to the overall dataset size. If the number of data errors decreases as the dataset grows, it signifies an improvement in data quality.
By defining and utilizing data quality metrics aligned with each dimension, organizations can obtain tangible measurements that reflect the quality of their data. This facilitates ongoing monitoring, evaluation, and the ability to identify areas for improvement.
Remember: the selection of data quality metrics that best align with your organization's requirements will depend on your specific use case. What matters most is to establish a data quality assessment plan to ensure that your data adheres to the necessary quality standards.
Putting data quality metrics into practice
Implementing data quality metrics in practice involves following a typical data quality assessment approach:
- Identify the specific portion of the collected public data that needs to be evaluated for data quality, typically focusing on information critical to your company's operations.
- Associate this data with the relevant data quality dimensions and determine the appropriate data quality metrics to measure them.
- Define ranges or thresholds that indicate high or low-quality data for each metric.
- Apply the assessment criteria to the data set, evaluating its quality based on the defined metrics and thresholds.
- Review and analyze the assessment results, deriving actionable insights and recommendations for improving data quality.
- Establish a periodic monitoring process by implementing automated checks and setting up alerts, such as email reports, to regularly monitor the quality of your data.
By following this approach, you can effectively track and maintain the quality of your data over time, ensuring its accuracy, completeness, consistency, validity, timeliness, and uniqueness.
How web scraping can ensure data quality
- Accurate and Timely Data: Web scraping allows businesses to extract relevant data from various online sources, ensuring access to accurate and up-to-date information. By regularly scraping websites for fresh data, organizations can maintain the timeliness of their datasets, which is essential for making informed decisions and staying ahead of the competition.
- Automated Data Collection: Web scraping enables automated data collection, eliminating the risk of human errors and ensuring consistent data quality. Automation ensures that the scraping process follows a predefined set of rules, minimizing inconsistencies and inaccuracies that can arise from manual data entry.
- Data Validation and Cleaning: Web scraping can incorporate data validation and cleaning techniques to improve data quality. Through web scraping, businesses can filter and validate the scraped data, eliminating irrelevant or erroneous information. This process ensures that only high-quality and relevant data is collected and utilized.
- Structured Data Extraction: Web scraping allows for the extraction of structured data from websites, which can enhance data quality and organization. By defining specific data elements to scrape and structuring the output in a standardized format, businesses can ensure consistency and uniformity in their collected data.
- SEO-friendly Scraping Practices: To maintain SEO hygiene, it is important to scrape websites respectfully and follow ethical guidelines. Adhering to robots.txt rules, using appropriate scraping intervals, and respecting website terms of service are some best practices to ensure SEO hygiene while web scraping. By maintaining a positive relationship with websites and search engines, businesses can mitigate the risk of being blocked or penalized.
- Compliance with Legal and Ethical Standards: Web scraping should always be conducted within the boundaries of legal and ethical frameworks. Respecting privacy policies, copyright laws, and terms of service is crucial to ensure data quality and maintain SEO hygiene. Compliance with these standards helps protect the interests of both the data providers and the organizations performing web scraping.
Let’s wrap up
In today's business landscape, data has emerged as a valuable asset, offering actionable insights, opening doors to new opportunities, and enabling companies to maintain a competitive edge. However, the true value of data lies in its quality. Therefore, businesses must prioritize the tracking and monitoring of data quality by implementing a robust data quality strategy. By doing so, organizations can ensure that the information they rely on is consistently accurate, reliable, and fit for purpose.